9. January 2025 | Interviews
Does automation replace experts or augment expertise? The answer is yes
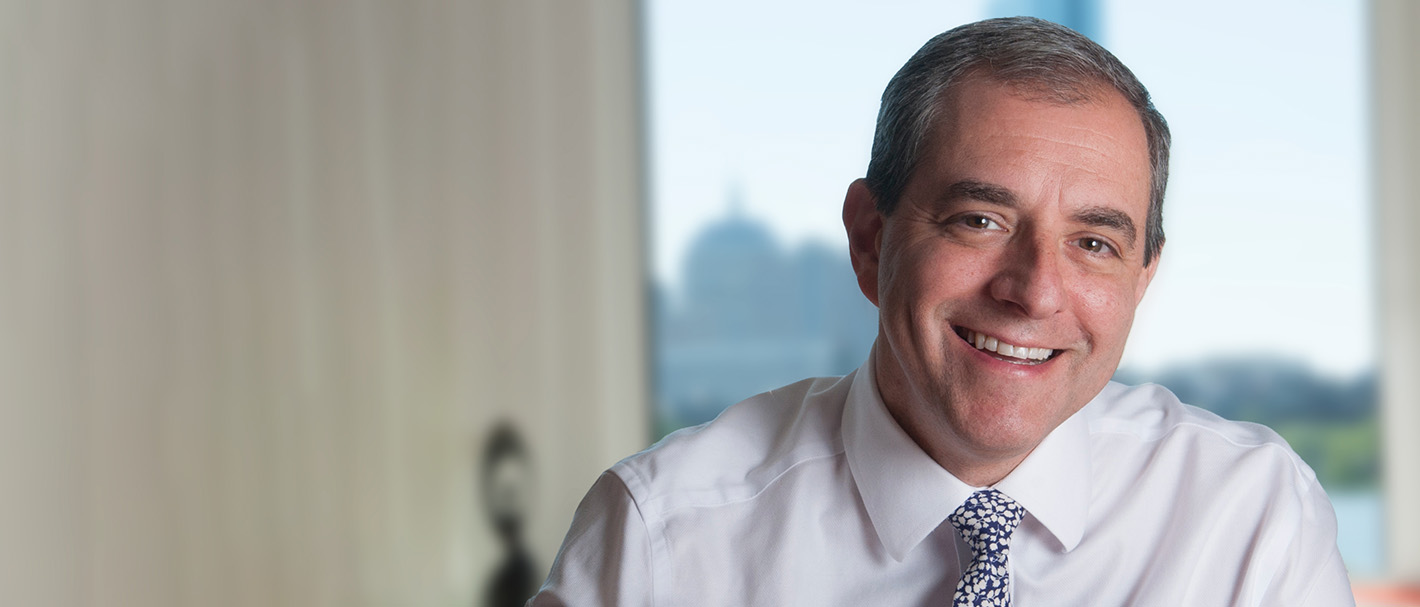
In your current research, you investigate how automation can simultaneously replace experts and augment expertise. Could you describe how technological change can have such seemingly contradictory outcomes?
If there is one thing on which Artificial Intelligence (AI) pessimists – or ‘doomers’ – and optimists agree, it is that AI will have transformative impacts on the labor market. But what these impacts will be is hard to fathom from either perspective because, ironically, both optimists and pessimists are driven by a shared conceptual fallacy, which I call the ‘exposure fallacy.’ This idea, pervasive among journalists, policymakers, and leading scientific publications, equates a job’s ‘exposure’ to AI – or computerization, or robotics, etc. – with its potential harms or benefits from that technology. In the eyes of doomers, ‘exposed’ occupations will inevitably atrophy in the face of technological advances. Conversely, optimists expect that those occupations will be ‘augmented’ as AI boosts their productivity.
But you don’t share either of these binary views?
It is not the case that employment and wages necessarily fall in ‘exposed’ occupations. More often, among similarly ‘exposed’ occupations, employment rises and wages fall, or employment falls and wages rise. What is missing from the pure ‘exposure’ idea is the crucial role played by human expertise. Expertise, meaning the knowledge and ability to perform specific tasks, is rewarded in the labor market under two conditions: first, the tasks that expertise enables are themselves valuable, e.g., data science, not card tricks; and second, the expertise is scarce, i.e., if everyone is an expert, no one is. Expertise is unlike other commodities because it is ‘downwardly’ but not ‘upwardly’ compatible. Expert workers can generally do inexpert work, but the reverse is not true. Plumbers can wait tables if the need arises, but table waiters cannot generally repair plumbing, even under duress. Expert labor is accordingly inelastically supplied, but inexpert labor is elastically supplied.
Technological changes that raise expertise requirements may raise wages without raising employment.
What role does expertise play in relation to AI’s impact on wages and employment?
The supply of expertise mediates how technologically-induced changes in task-demands affect the set of workers who are qualified to perform newly reconfigured jobs, and hence their pay and employment. Because both experts and non-experts can do inexpert work, technological changes that reduce expertise requirements in an occupation may lower or fail to raise wages in that occupation even as they raise occupational productivity and employment. Technological changes that raise expertise requirements, however, may raise wages without raising employment because inexpert workers cannot quickly flood into expertise-intensive jobs. In both cases, the number of tasks automated is of less importance than the expertise requirements of the tasks removed. If an occupation’s expert tasks become automated, occupational productivity will rise, but the remaining inexpert work will be accessible to any worker. This may lead to a seemingly paradoxical case of rising employment and falling wages. Conversely, if an occupation’s inexpert tasks are automated, productivity will again rise, but fewer people may be qualified to perform the tasks that remain, leading to the reverse case of rising wages and falling employment.
Given your earlier work on the impact of technological change on skills-based labor demand, how is modern technological change similar to or distinct from technological change in the past?
The ‘Task Model’ has served as the template for the exposure-based approach to predicting the impacts of AI. Colleagues Frank Levy and Richard J. Murnane and I introduced the task model in a 2003 paper, and Daron Acemoglu and I provided a modern theoretical formalization of this conceptual apparatus in 2011. Many have built on this foundation, including David Dorn and myself in 2013, Maarten Goos, Alan Manning, and Anna Salomons in 2014, and Daron Acemoglu and Pascual Restrepo in a copious collaboration beginning in 2018. The task model conceives of an occupation as a set of tasks that must be performed to accomplish an objective, and it asks which of these tasks will be accomplished by workers and which by computers. As crafted in 2003, this framework recognized that so-called routine tasks – tasks that are easily codified into formal rules and procedures – were at that time far better suited to computer-based automation than tasks requiring judgement, reasoning, and management of others, i.e., non-routine cognitive tasks, or tasks requiring dexterity, flexibility, and common sense, i.e., non-routine manual tasks. Occupations that specialized in such routine tasks, such as production-, clerical-, and many administrative support-positions, were likely to be directly affected by computerization. Empirically, the share of an occupation’s tasks which could be automated was quantified as its ‘exposure’.
During the computing and robotics revolution of the last four decades, exposure meant task displacement and simplification of work.
The Task Model has been broadly applied by economists to analyze past technological breakthroughs such as computerization and robotization and is increasingly used to make predictions about how AI will impact the labor market. During the computing and robotics revolution of the last four decades, exposure meant task displacement and simplification of work: the expertise of many middle-skilled production and administrative workers was made redundant by automation; non-college workers who would have taken such jobs in the pre-computer era were instead shunted into relatively inexpert jobs in low-paid, manual task-intensive services such as food service, cleaning, security, recreation, and non-credentialed care services.
This mechanism, specific to the prior era of procedural computing, should not simply be cut and pasted onto the AI revolution. As I argued last year, AI is best-suited at present to performing non-routine cognitive tasks, those requiring judgement in open-ended decision-making tasks. How this newly-minted and rapidly improving machine capability affects demand for human expertise will differ in the present era. While in both eras, the relevant question is how demand for expertise is reshaped by new tools, the answers will differ because the forms of expertise that are either automated or complemented are distinct.
Research seems to indicate that technology-driven changes in the European labor market differ from, or are delayed compared to, those in the United States. Could you discuss how wage-setting institutions and labor-market regulations in Europe compare to those in the United States in mitigating or offsetting the negative impacts of technological change on the labor market?
It’s no secret that the U.S. does very little to help displaced workers gain new skills and make successful transitions into new employment. Adding injury to insult, the U.S. unemployment insurance system is stingy and, for many workers, difficult to access. And of course, the ability of U.S. labor unions to offer additional supports and protections is severely atrophied after decades of declining union strength. I think many European countries are more humane, more forward-looking, and more successful in buffering the economic cost of job loss and in supporting successful transitions. The 2023 paper by Bertheau and colleagues “The Unequal Consequences of Job Loss across Countries,” makes this point compellingly. The consequences of job loss are never positive. But the size of the economic losses and the speed and success of transition to new employment depends greatly on labor market institutions.
I think many European countries are more humane, more forward-looking, and more successful in buffering the economic cost of job loss and in supporting successful transitions.
Given your earlier work describing how shifts in the age-structure of occupations help to explain the relationship between technological change and changes to employment, can you talk about the impact of current automation trends on middle-age or older workers, especially those who have been displaced?
The demographic challenges which industrialized countries face are significant. Across most of Europe and Asia – including China –, the number of prime-age workers, ages 20-64, is set to decline by 5 to 40 percent over the next 35 years. Simultaneously, the number – and the share – of adults ages 65 and over will substantially increase. This means that a shrinking set of workers will need to support the hard-earned, and well-deserved retirements of many older adults. These younger workers will need to be significantly more productive to make this transition successful. Fortunately, current cohorts of workers are the most educated in history, and they are working longer than prior cohorts because they are healthier, do less physically demanding work, and because educated workers generally find their jobs more enjoyable – both because the work is less physically demanding and because it is often more intellectually rewarding.
Older workers will be badly needed, and they are generally faring relatively well.
The slowdown in retirements that has resulted from these changing demographics is, on the one hand, positive for maintaining a larger labor force despite an aging population. On the other hand, evidence from papers by Paul Mohen, Nicola Bianchi, amonst others, suggests that the slowdown in retirements has hindered the upward mobility of younger generations into high-skill, high-ranked positions. There is no evidence that I know of that this is slowing economic growth in aggregate, but it’s surely unhelpful to the young. This set of circumstances – specifically, the shortfall of younger workers relatively to older citizens – makes me less worried about the impacts of automation on the workforce than I otherwise might be. Older workers will be badly needed in the workforce and, due to their high educations and relatively good health as compared to earlier generations, they are generally faring relatively well.
Although I’m generally optimistic, if the pace of technological upheaval accelerates – as it might – then older worker may in fact be more vulnerable.
At the same time, recent evidence from Battisti, Dustmann, and Schönberg finds that when organizations undergo technological and organizational changes, older workers are less likely to be retrained and more likely to exit the labor force. Thus, although I’m generally optimistic that older workers in industrialized countries like Germany are faring relatively well, if the pace of technological upheaval accelerates – as it might – then they may in fact be more vulnerable.
As we look to the future, what lessons can we draw from the past about the role of education and training in helping workers maintain access to high-quality work throughout their working lives?
Perhaps the most important lesson of the 200 years of evidence we have on skills and earnings is that public investments in education have been the most successful, reliable, and broadly forward-looking policies we’ve deployed to enable workers to succeed over their lifetimes, as I found with Claudia Goldin and Lawrence F. Katz in a 2020 paper. Most of that education occurs prior to labor market entry; however, and in general, investments in training and education later in life have a mixed track record. This is an argument for building foundational skills early in life. We also know that some adult education programs can be successful. Lawrence F. Katz, Jonathan Roth, Richard Hendra and Kelsey Schaberg discuss in a 2022 paper what types of programs succeed in the U.S. Anders Humlum, Pernille Plato, and Jakob Munch show even more promising evidence from Denmark.
Public investments in education have been the most successful, reliable, and broadly forward-looking policies we’ve deployed to enable workers to succeed over their lifetimes.
What else is needed to accompany the AI transition?
Although many believe that Artificial Intelligence will make highly educated workers less needed in the future, I wouldn’t place my bet there. The track record of human capital investment generally, and higher education broadly, is too formidable to give up on that tool before we see clear evidence that it no longer pays off – and I don’t think such evidence will be coming soon. At the same time, I think that we’ll need to get better at helping adults to “change tracks” as some types of work are rapidly reshaped by advancing technologies. I believe that the technology itself, if we use it well, will enable us to build better tools for supporting these transitions.
Thus, the proper policy focus is not either skills or insurance. It’s both.
At the same time, skills are not enough. We need a robust social insurance system to assist and support workers and others facing rapidly changing labor market circumstances. Thus, the proper policy-focus is not either skills or insurance. It’s both.
More about Professor David Autor
David Autor is a Professor and Associate Department Head of the Massachusetts Institute of Technology Department of Economics. He is also a Faculty Research Associate of the National Bureau of Economic Research and Editor in Chief of the Journal of Economic Perspectives (published by the American Economic Association), and has served on the Board of Editors at the American Economic Journal: Applied Economics and the Journal of Labor Economics.
Image: Peter Tenzer Photography
DOI: 10.48720/IAB.FOO.20250109.01
Winters, Jutta; Latner, Jonathan (2025): Does automation replace experts or augment expertise? The answer is yes, In: IAB-Forum 9th of January 2025, https://www.iab-forum.de/en/does-automation-replace-experts-or-augment-expertise-the-answer-is-yes/, Retrieved: 15th of January 2025
Diese Publikation ist unter folgender Creative-Commons-Lizenz veröffentlicht: Namensnennung – Weitergabe unter gleichen Bedingungen 4.0 International (CC BY-SA 4.0): https://creativecommons.org/licenses/by-sa/4.0/deed.de
Authors:
- Jutta Winters
- Jonathan Latner